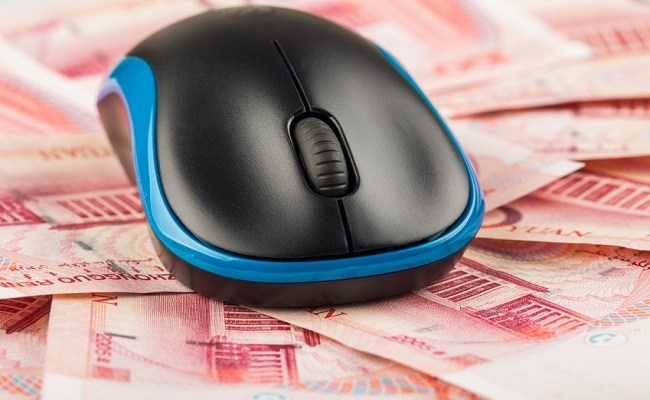
By Henry Hing Lee Chan
Can Big Data Detect “Character”?
One of the most famous quotes of the legendary financier J. P. Morgan was of his criteria for extending loans: “The first thing [in credit] is character … before money or anything else. Money cannot buy it … A man I do not trust could not get money from me on all the bonds in Christendom. I think that is the fundamental basis of business.”
This quote of J. P. Morgan on the unique role of character in the 4Cs of credit — character, capacity, capital and conditions — is a poignant reminder of the importance of human judgment in determining whether a loan will end up being repaid or go into default.
The simplest way to define these four Cs is as follows: Character is honesty in the simple words of J. P. Morgan. Capacity reflects the ability of the business to pay its bills. Capital reflects whether the borrower has the financial resources to repay the loan. Conditions reflect the external factors surrounding the loan under consideration.
In the last few years, the emergence of peer-to-peer (P2P) lending platforms seemed to convince many that the traditional four Cs in loan evaluations can be handled more efficiently by machines rather than humans. The P2P platform created easy-to-use websites that matched consumers and small businesses with individuals or investors looking to lend money. These P2P platforms are freed from the cost of brick-and-mortar branches as well as banking regulations that require reserves. These inherent cost advantages allow P2P platforms to have higher margins. As such, they have grown quickly in the past couple of years. Loans can be processed in days instead of months under the traditional banking system. They are celebrated as new financial disruptors that are set to transform the financial industry into a paperless, frictionless, and transparent world and will render the old banking system obsolete.
However, the recent crackdown of P2P platforms in China revealed the structural defects in the original setup. The industry watch group, WDZJ, found that up until April this year, 1598 P2P platforms had either shut down, defaulted on repayments, or their company officers simply vanished. This constitutes almost 40 percent of the P2P companies tracked. The crisis in P2P loans happened not only in China; the US’ celebrated Lending Club also ran into a serious credibility crisis in May and saw its price drop more than 50 percent between May 1 to 16 after revelations of operational improprieties forced the resignation of its founder CEO.
One basic issue with P2P is the behavior modification of the borrower once the loan is obtained. While computer-based “big data” can capture three of the four Cs relatively well — capacity, capital, and conditions — predicting changes in character after the borrower gets the loan remains a challenge. His past credit record might be good but his behavior can easily change if a bigger sum suddenly come to him easily. Of course, the situation will be worse if potential borrowers present falsified data to game the computer algorithm designed to capture character aspect of the four Cs.
In view of P2P lending problems in China and the US, computer-based credit evaluation systems have apparently failed to solve the question of character in the 4Cs of credit extension. Whether a computer algorithm can one day solve this issue and herald in a new age of finance remains an unknown. As of now, it seems human credit gatekeepers will still be around for a long time.
Leave a Reply
Your email address will not be published. Required fields are marked *